While data can be invaluable in identifying loss patterns, it’s important to remain mindful of its most critical vulnerability: data corruption, also known as the principle of “GARBAGE IN, GARBAGE OUT” (GI-GO).
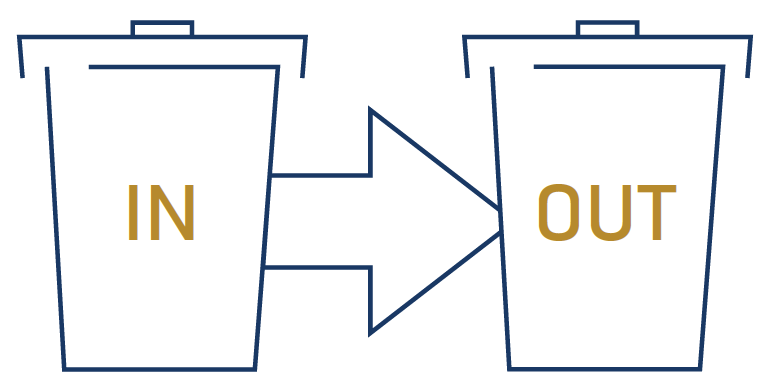
It is essential to ensure the accuracy of the information used for critical decision making to effectively manage your overall cost of risk. Clean, reliable data is vital for making informed and optimal choices. Frequent and regular inspections of your loss data collection method and the data itself should be a standard operating procedure to ensure you maintain clean data.
Key questions to consider include:
- Is your data collection method effective and reliable?
- Are you able to use it to drive change and when you audit, is it accurate?
- Are there additional fields you should include to capture critical information?
- Is your data unable to answer any questions?
- Do your drop-down menus need updating to reflect new or relevant options?
- Are you collecting fields that are outdated, unnecessary, or not being analyzed as part of your loss control efforts?
Conducting regular variance checks on your data can help you avoid significant downtime in the future. Employ a combination of methods, including:
Conduct a Random Full Loss File Audit.
- Select a reasonable sample size of files and pull the original documentation.
- Review and compare these records against the data entered into your Risk Management Information System (RMIS) to ensure accuracy.
- Review post-event investigation documentation and cross-check it with the RMIS system records.
- Evaluate root cause analysis documentation to confirm whether managers or supervisors implemented corrective actions to prevent similar incidents.
- If you uncover discrepancies between reported information and actual events, identify the root cause of the variance and address it promptly.
Review the Loss Data in Excel While Sorting by Key Fields and Look for Consistency in Entry and Coding.
- Are all the fields being populated?
- Is there an overuse of generalization or unknown descriptions?
- Does the event description align with the coding that is entered?
- Does the employee demographic information match up with that specific employee?
- Be mindful of instances where individuals may enter placeholder or incorrect information to get the report submitted. This saves time when someone doesn’t have the required field information immediately available, like hire dates or job functions.
- Generalization codes often include Not Otherwise Classified (NOC), Human Action, Unknown, and Not Applicable (N/A) instead of the correct information.
- If your system is linked to your HR database, verify that all codes are being transmitted accurately. Check whether HR has added new job titles that aren’t reflected in your current tables and update your system accordingly to maintain alignment.
If You Have a Third-Party Administrator (TPA) or Carrier Where the Claims Persons Are Responsible for Coding Certain Fields that Could Be Valuable to Your Analysis, Ensure Those Fields Are Being Populated Accurately.
- Initial coding is sometimes handled by less experienced administrators, so it’s essential to review and verify the accuracy of this data to avoid potential issues.
These are some of the most useful, common codes that may be applied by the carrier or TPA:
- Part of body
- Nature of injury
- Source of injury (not all TPAs or carriers)
- Cause of injury
-
- Accident type (backing, intersection, hit by other, rear end collision)
- Employee demographic information
Inspect Your Output
You can build the world’s greatest reporting system to capture your losses, but if you don’t regularly inspect the results, your data will inevitably include inaccuracies or “noise.” Data may be missing, and identifying and filling those gaps will be crucial. These gaps can come from the employee failing to provide the information or a section being skipped by a supervisor in error during input. Regardless of the cause, it’s important to retrieve and input the missing information. To prevent these issues, it’s best to design your collection methods to minimize the possibility of omitting critical information.
Working with Missing Data
Can some of the missing information be derived from the fields you already have?
- Audit Scenario
During a data audit, you discover that supervisors are consistently skipping field 24—in this case, a critical question for understanding your losses.
- Solution
Analyze the surrounding questions and build logic into your collection system to automatically calculate the missing information. For instance, use the data from fields 23 and 12 to generate an accurate response for field 24.
- Field 12: What time did the incident occur?
- Field 23: What time did employee start their shift?
- Field 24: How many hours into the shift was the employee when the event occurred?
To ensure high-quality data, maintain ongoing communication with your end users and identify opportunities to improve your collection methods. Put yourself in their shoes—if the system is overly complicated or cumbersome, users are likely to develop workarounds, resulting in inaccurate or incomplete data that won’t be actionable.
Data drives decisions; by ensuring its accuracy, your outcome is more likely to be successful.
author
Jamie
Hofer
Risk Control Analyst,
Client Advantage